Leveraging Artificial Intelligence for Social Work
Author(s)
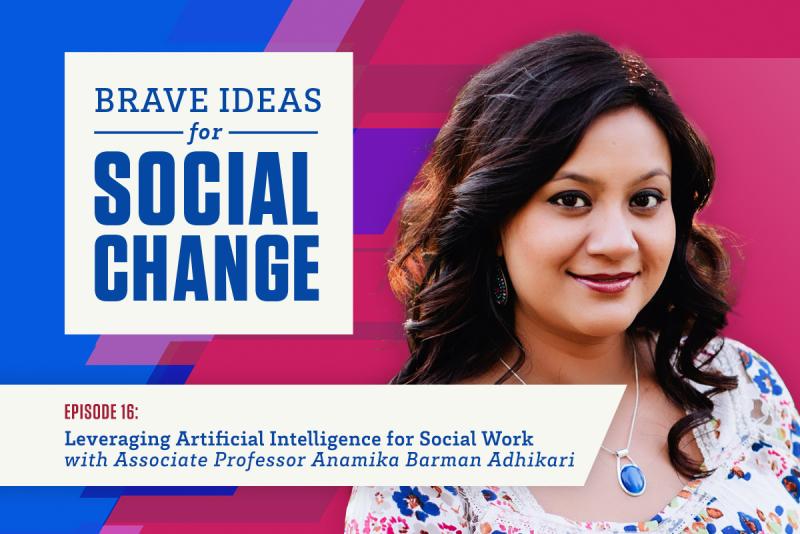
Brave Idea: Associate Professor Anamika Barman Adhikari is one of only a handful of U.S. social work scholars with expertise in using social network methodologies and advanced computational methods such as machine learning in research. Her research interests are broadly centered on understanding the social–contextual determinants of risk and protective behaviors among vulnerable populations, including minority youth and youth experiencing homelessness. She examines how social networks and norms shape risk and protective factors for marginalized populations, explores digital practices among youth experiencing homelessness and other minority youth and young adults, and develops and disseminates programs that use innovative technology to increase social connectedness and preventive behaviors. She advocates for the use of artificial intelligence (AI) and other technological and methodological advances in social work research and practice. Barman Adhikari is driven by the belief that social workers are uniquely positioned to help guide the development of AI in fields beyond social work.
Listen:
Resources:
The Grand Challenge to Harness Technology for Social Good
ChatGPT for Social Work Science: Ethical Challenges and Opportunities
National Association of Social Workers: “AI and Social Work”
Artificial Intelligence in Social Work: Emerging Ethical Issues
Transcript:
Dean Henrika McCoy:
Hello, I'm Dr. Henrika McCoy, professor and dean of the Graduate School of Social Work at the University of Denver. Welcome to episode 16 of the school's Brave Ideas for Social Change podcast series, which draws on GSSW faculty expertise for fast moving discussions on emerging research, practice and policy innovations disperse social change.
I've been following the podcast since it launched in 2021 and it's GSSW's new dean I'm so excited to be behind the microphone now facilitating these important conversations and living my dream of hosting a podcast
Today's guest is associate professor Anamika Barman-Adhikari, one of only a handful of US social work scholars with expertise and using social network methodologies and advanced computational methods such as machine learning and research. Her research interests are broadly centered on understanding the social contextual determinants of risk and protective behaviors among vulnerable populations, including homeless and minority youth. She examines how social networks and norms shape risk and protective factors of marginalized populations, explores digital practices among youth living without homes and youth and young adults of color. She also develops and disseminates programs that use innovative technology to increase social connectedness and preventive behaviors.
Dr. Barman-Adhikari. I am so looking forward to learning more about this deciding area of research. I know nothing, so I am prepared to be a student and learn just like our audience.
Anamika Barman-Adhikari:
I appreciate that and thank you so much for the kind introduction.
Dean Henrika McCoy:
Harnessing technology for social good is one of the grand challenges for social work that is focused on creating a stronger social fabric, notably using technology in new ways, especially rapidly evolving areas such as artificial intelligence is one of those areas many of us are unfamiliar with. Would you start by sharing with our listeners what are artificial intelligence and machine learning?
Anamika Barman-Adhikari:
Of course, that's a great starting point. When I started using AI and machine learning in my own work, I had no idea what they meant. The only thing that I could think of is The Terminator. Most of us have watched that movie about the time traveling, very human-like robot, and I think there's a lot of confusion about what AI and machine learning means. So I'm glad we are starting out with that question. So back to the question about what is artificial intelligence and machine learning? These terms are often used interchangeably, but they have very different meanings. So think of AI as the big umbrella. It refers to broader concept of creating intelligent machines that can mimic human processes such as learning, reasoning, problem solving, and perception. And it could include a variety of things like robotics, computer vision, natural language processing, and machine learning to name a few.
So as you might have figured out, machine learning is a part of AI, so it's a specialized field under that bigger AI umbrella. And what it involves is creating algorithms and models that enable computers to learn from and make predictions from data. So let me give you an example of an algorithm that's easy to understand even if you're not familiar with the topic. I mean most people nowadays use social media, I use Facebook, Instagram, et cetera. And we have probably at some point wondered how does social media know exactly what ads to show us? And they're very accurate in showing those ads almost like they're reading our minds, right? They are almost reading our minds, not literally though. They often do it by tracking our digital footprints. So when we browse our feeds and we like post or we click on links, they are tracking all that activity.
So the algorithms use all this data, use all this information to figure out what kind of products or services we might be interested in. For instance, if you're always liking posts about outdoor gear, hiking, the system might predict that you're interested in these things. So it might show you ads for hiking boots, camping gear, and even travel packages for maybe a nature trip that you've always wanted to take. And this is just one example. AI is everywhere these days from recommending movies on your Netflix feed maybe and self-driving course, navigating busy streets. So AI is a really powerful tool and any tool, it can be used for good or bad. And social workers really need to understand AI and its potential. And I'm so glad we are having this conversation today.
Dean Henrika McCoy:
So how would you say these sorts of technologies are already present in social work, research and practice?
Anamika Barman-Adhikari:
We are still in the very early stages, but AI and machine learning are starting to become more widely used in social work, both in research and practice. Before we dive into this question, I should mention that we shouldn't just use AI because it's new or exciting. It's important to be thoughtful about how and why we use this technology. As social work researchers and practitioners, we need to ask ourselves, what does this tool offer that our established methods cannot achieve? It's important to remember that AI cannot replace the human touch, which is at the heart of social work. So talking about a few cases where AI can help social workers do their jobs more efficiently and also more effectively. Let's start with an example. I work in the area of youth homelessness and predicting who might experience homelessness in the future can help us provide important information about who we should target for services.
Typically, social workers use historical data, interviews, community assessments to identify at-risk young people or their families. And while these methods are partially effective, they can be time-consuming and may not always capture emerging patterns or subtle risk factors. And AI can really enhance this process by analyzing large data sets quickly and pretty comprehensively. So an AI model might analyze data from school records, social service histories, family background to identify students who are exhibiting any signs of distress or instability. By flagging those individuals early, our hope is that social workers can initiate targeted support services.
And not only that, one of the advantages of using AI is that it can continuously integrate that data so that as new data becomes available, it can make real-time adjustments to these models and provide up-to-date insights about who we should be targeting for services. So AI is not about replacing social workers, but making their jobs easier and more efficient.
Dean Henrika McCoy:
Okay. I think social workers will be relieved to hear that. So you've been using innovative technology-driven methodologies in your own research. Is that right?
Anamika Barman-Adhikari:
That's correct.
Dean Henrika McCoy:
Could you tell me a little bit about that?
Anamika Barman-Adhikari:
Of course. So I have been using innovative tech-driven approaches to better understand youth homelessness and their unique needs. And it ties to my earlier point about being mindful why we use AI. There's always a purpose beyond just novelty. And to do that, let me share a bit of context about my work before explaining how I have used AI, especially natural language processing in one of my projects. So I focus on young people experiencing homelessness, and they're a highly marginalized group. Not only are they hard to reach, but they're also very difficult to engage in research and intervention just because they're so transient.
My main interest is in their social relationships, who they interact with, like their peers, parents, siblings, caseworkers, foster family, and how these connections influence their health and behavior. So when I was doing my dissertation research and I was interviewing, surveying these young people about their social relationships, one of the patterns that kept on emerging is that the young people who stayed connected with people outside of their street networks like family or siblings, tended to fare much better than young people whose relationships were primarily street-based.
And when we look deeper into that data is that we realize that many of these positive relationships were being maintained via technology, like social media and cell phones. So that discovery led me to dig deeper into how technology might be a critical tool to understand these young people better, and especially because they're so hard to reach through regular research techniques. So when I arrived here at DU, I applied for and received a grant to analyze these young people's Facebook feeds. So we scraped their Facebook feed and to explore what they were talking about and how. So I'll admit, when I first started this data, I was very naive. I didn't anticipate the volume of data we would be gathering. So we had close to 200,000 posts from 160 young people.
And it quickly became clear to me that manual analysis of that data is not possible. So that's when I started reaching out to collaborators, especially in the School of Information Sciences here at DU. And thankfully I got connected to these two faculty, wonderful faculty members who were willing to collaborate with me. And that's when they said, "Hey, let's use natural language processing, which will make this task much more efficient." So natural language processing for people who don't know is basically AI's way of understanding human language. So in my research, it was a game changer for analyzing those 200,000 posts because what it did is it allowed us to spot patterns, themes, and even the emotions behind these posts, something we could never do manually. So the computer was doing that. And what natural language processing does very well is that it doesn't only do topic modeling, so what are common across these posts, but they also do something called sentiment analysis.
So understanding whether the tone is positive or negative or neutral, and even the feelings expressed, are they expressing hope? Are they expressing frustration? Are they expressing loneliness? And this is especially important when we are trying to get a sense of what is going on in the minds of these young people who might not be willing to share that in a survey or in an interview because of social desirability reasons. So for example, in one of my papers that use this data, what we did is that we looked at these conversations, we use sentiment analysis to get a sense of the emotional tone in their post, while also examining their interactions with their peers and other people on social media. And what our goal was to do is to predict substance use behavior from that information.
So to do this, we apply advanced techniques using natural language processing that allowed us to accurately predict patterns about 77% of the time. And as you might know, with regular regression, we probably capture 20 to 25% of the variance. So AI and NLP can be really used very well together to get more accurate and actionable insights.
Dean Henrika McCoy:
Wow, that is amazing. The possibilities are quite incredible, aren't they?
Anamika Barman-Adhikari:
Absolutely. I have often thought about how AI and social work can come together. So I've been picturing this, right, a future where social work is seamlessly blended with advanced AI and data analytics. So for example, if you could use AI to spot early signs of substance abuse or mental health issues based on data such as social media data, data from health surveys, this to really allow us to step in earlier and prevent the problems from getting worse. And AI, one of the beneficial aspects of AI is that it could also enable us, especially in the world of social work, to customize interventions.
So for example, if we are working with clients who have experienced trauma and need therapeutic interventions, AI could analyze data from previous cases. AI could identify clients with similar trauma backgrounds and see what specific CBD technique or what specific mindfulness technique has worked for people with similar profiles. And we could customize interventions based on that data. So the possibilities are endless, but none of these ideas are just in place yet, but we can always dream.
Dean Henrika McCoy:
So what would you say are some of the issues that impede progress?
Anamika Barman-Adhikari:
Well, resources, I think social work often struggles with funding, as we all know as social workers partly because we focus on the most marginalized communities. And even though social work provides immense value to society, we don't want, it doesn't translate into real money, our revenue. So the struggle is about securing resources needed for these innovative projects. Having that kind of skill is not cheap. And how do we provide nonprofit organizations or even researchers, the resources they need to carry on these expensive endeavors.
Dean Henrika McCoy:
So in addition to the many potential positives of this technology, what would you say are potential pitfalls that social work researchers and practitioners should be aware of?
Anamika Barman-Adhikari:
Well, there are quite a few, as if any technology there are good and bad. But I think one of the biggest problems with algorithms is that they can be kind of like black boxes, which means that they're not easy to understand. It's trying to figure out what's happening inside a complicated machine without being able to open it up and see all the parts. So let's give you an example. So for example, Allegheny child welfare system recently, not recently, about three years ago, used this, developed this tool called the Allegheny Family Screening Tool. And it was created to predict the likelihood that a child might be placed in foster care within two years after a family is investigated. And it used a lot of detail, personal data like child welfare history, birth records, Medicaid data, substance abuse and mental health records that even jail and probation records. So they had this access to this fast throwback data.
And the tool gives a risk score from one to 20 with higher numbers predicting higher risk. However, an audit revealed a very significant issue. If the tool made decisions on its own, it would have recommended investigating two thirds of Black children compared to about half of children from other racial groups. And this points to potential bias in how the algorithm assesses risk. So since the historical data points to over representation of Black children in the child welfare system, that's what the algorithm is picking up. So I always tell this to people that your algorithm will only be as good as your data.
Dean Henrika McCoy:
So that's I think an excellent example related to social work, but problems related to equity and justice could really show up on a grander scale. Is that true?
Anamika Barman-Adhikari:
Absolutely, yes. I think a great example is what happened with Amazon's recruitment system. So the idea was for this AI tool to automate evaluating job candidates based on how well they fit different roles. And the AI learned by an analyzing resumes from past applicants. However, since women had historically been underrepresented in tech roles even today, the system started favoring male candidates assuming they were better suited. So it ended up unfairly downgrading CVs from a woman. Amazon tried to correct it, but they eventually scrapped it because people were really getting concerned about it. They scrapped it in 2017. And again, this shows that AI can reinforce existing societal biases because our data reflects that, right? So you always have to check your data, it's garbage in, garbage out.
Dean Henrika McCoy:
So this technology is here to stay, and I expect we'll continue to see it advance by leaps and bounds. So how does social work catch up and then actually keep up?
Anamika Barman-Adhikari:
Yeah, this technology is advancing quicker than anyone, and people are getting really concerned about AI and its potential, the potential it has on everybody's future. But unfortunately with social work, we are lagging behind very significantly in using AI. And I'm not saying it's a good or a bad thing, but I think social work can be made more efficient using AI. And while I don't have all the answers, I think a few ideas might help us catch up and keep up ongoing education and training in these new technologies. So for example, the University of Southern California has a course where computer science and social work students come together to tackle complex social issues. And the point isn't to turn social workers into computer scientists or vice versa. That's not possible. Instead, it's about building common ground, a common language, and do more interdisciplinary work.
Also, we need to be smart about forming partnerships, especially when it comes to research. I could have never done my research using AI if not for my collaborators in computer sciences or information sciences. So we just need find new ways of connecting, maybe organizing more interdisciplinary meet and greets across departments. And that's how I met my collaborators.
And third, and my final point, we need to be more assertive in showing up in these spaces. This isn't just about AI, it's more of a bigger issue that we always faced as social workers, where social workers and scholars are often misunderstood or not fully appreciated. And people tend to have this very narrow, sometimes negative view of what we do overlooking the larger impact that we can make. And when it comes to AI, especially, I think we have a huge role to play because of our focus on ethics, on social justice and supporting vulnerable communities, especially since we know AI can perpetuate biases that we see in society. So we really cannot wait for an invitation to this conversations. We need to step up and get involved.
Dean Henrika McCoy:
Okay, I love that call out to our fellow social workers. So what can and should social work practitioners be doing in their own work, and what do you think they need to look out for?
Anamika Barman-Adhikari:
Great question. I think we can take several important steps in our own work, and especially practitioners. A lot of child welfare departments across the country especially are starting to integrate AI. So staying informed about AI, being good consumers of that knowledge is very, very important. I think it's great to know how these tools can improve outcomes, but we also need to be very mindful of the potential risks like bias in algorithms or privacy issues especially.
Also, we need to be very critical in thinking about how we are using technology. Are these tools really genuinely enhancing the services we provide or are they just adding unnecessary complexity and are they really addressing the needs of the communities we work with? Or they could reinforce existing inequities. And I think we should use these tools as helpful guides, but we should always trust our own gut and our own instance when it comes to an understanding of our client's unique situation and not AI, let AI dictate that.
Dean Henrika McCoy:
Well, I want to thank you. This has been such an interesting and thought-provoking conversation and thank you so much for all of your efforts to advance this work.
Anamika Barman-Adhikari:
Thank you so much for having me.
Dean Henrika McCoy:
You're welcome. So for more conversations like this, please subscribe to our Brave Ideas for Social Change podcast. You can learn more at socialwork.du.edu/change. And thank you for joining us today.