Preventing Youth Suicide
Prof. Anthony Fulginiti uses machine learning to study suicidal behavior among youth experiencing homelessness
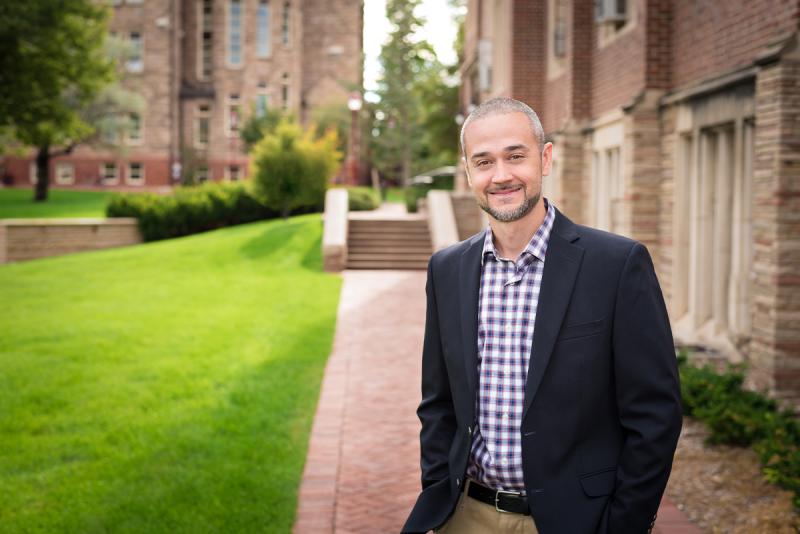
Can machine learning help to prevent suicide? University of Denver Graduate School of Social Work (GSSW) Associate Professor Anthony Fulginiti thinks so. He applies social network and machine learning approaches to suicide prevention research, aiming to relieve suffering and prevent suicide among vulnerable populations, including youth experiencing homelessness (YEH).
In one of several projects underway, Fulginiti is working to improve gatekeeper training — a widespread approach to suicide prevention — by identifying the people in a social network who are best positioned to deliver peer suicide prevention interventions. Most often, gatekeeper training is delivered in school settings. But YEH are often disconnected from school, and thus, “These young people are disconnected from many evidence-based suicide prevention practices,” Fulginiti says. He is working to find ways to customize gatekeeper training to YEH and the service settings where those youth interact most, such as drop-in centers.
To do that, he plans to try to recruit young people in a transitional housing facility and map help-seeking behaviors in their social networks. Using artificial intelligence, he will then determine who within the networks are the natural “gatekeepers” — the individuals who are well connected and influential and therefore most likely to be able to effectively deliver a peer-based suicide intervention. Fulginiti also will conduct focus groups with those young people and service providers to build a gatekeeper approach that best fits their unique strengths and needs.
“We want to take these evidence-based practices and adapt them so they can be equitably distributed — so these young people can benefit from these evidence-based practices like those who are better resourced,” Fulginiti says, noting that data collection for the project should wrap up by the end of the academic year.
Predicting Suicidal Behavior
Despite extensive research, the prediction of suicide remains extraordinarily difficult, Fulginiti says, noting that one possible reason is that the influence of social factors — including social network members’ behaviors and beliefs — on suicide risk have largely been minimized or ignored.
Fulginiti is changing that picture, however. In a recent study published in the Journal of the Society for Social Work and Research, Fulginiti and GSSW alumna Jennifer Wilson (PhD ’21), along with several other collaborators, used machine learning to predict suicidal ideation and suicide attempts among YEH, who are particularly vulnerable to suicide. It is the first known study to leverage machine learning to better understand and predict suicidal ideation and suicide attempts among YEH.
In addition to individual suicide risk factors such as trauma and depression, the team identified several social network factors — such as hard drug use and tolerance for risky behavior among peers — that were associated with suicide risk among YEH. The clinical risk profiles the team developed may eventually be useful for suicide risk screening and guiding targeted suicide interventions for YEH.
“Our work demonstrates that networks matter. We found that numerous social network characteristics were prominent correlates of suicidal ideation and, to a lesser extent, suicidal behavior. In fact, more than half of the factors identified as being important in our [decision tree] analyses were related to the network environments of YEH,” Fulginiti and his coauthors wrote. “There is a paucity of social work scholarship about suicide among YEH, whose suicide risk may be rooted in their profound social disadvantage. We showed that the norms operating in the environments of YEH deserve more attention in the context of suicide prevention programming. Social network assessments and interventions may be a promising way to augment individual-level strategies.”
Understanding Firearm Violence
Fulginiti also is a co-investigator in a new three-year study funded by the Centers for Disease Control and Prevention. The study will examine the personal, social network and environmental contributors (such as neighborhood characteristics) to firearm violence among YEH. Fulginiti and researchers at the University of Missouri, University of Southern California, and University of California Irvine are recruiting 750 YEH in St. Louis and Los Angeles. They aim to develop a better understanding of firearm access and the drivers of firearm violence — particularly in the context of intimate partner violence and suicide.
“Firearm violence is understudied among youth experiencing homelessness, and firearm prevention programming is underdeveloped in this population,” Fulginiti says. “We don’t even know much about the contributors to firearm violence in this group; you have to look at that before you can build programming to prevent it.”
As part of the project, the team plans to use Google Street View to understand the characteristics of the neighborhoods (and even specific blocks) where YEH are living and the influence of those characteristics on firearm risk. “This will help us think about and design violence risk strategies in a much more precise way, customized to the population,” Fulginiti says, noting that a broader benefit of the project is to showcase a novel use of free technology that local agencies could use independently.
“People experiencing homelessness tend to be an invisible population, and if you’re not shedding light on the problem of firearm violence, it’s all too easy for it to be ignored and for the needs of that group to be ignored,” Fulginiti says, noting that receiving a substantial federal grant to study firearm violence among YEH acknowledges that the needs of this population matter. “Our hope is that this study is a meaningful step toward doing something about it.”