Preventing Substance Abuse
GSSW assistant professor is studying use of artificial intelligence to improve outcomes of substance abuse interventions for homeless youth
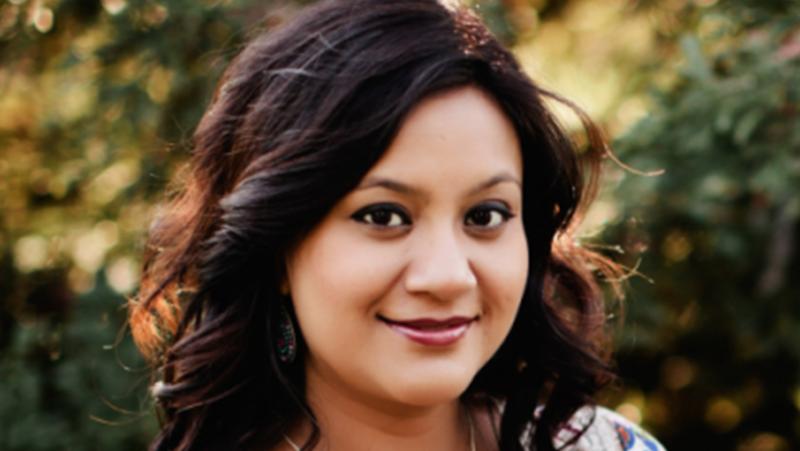
Group interventions are a common approach to substance abuse treatment for youth. The aim is to reduce high-risk behaviors through positive social influence. Sometimes, though, abusive behaviors actually increase when at-risk individuals come together to discuss risky behaviors, derive status from those behaviors and then engage in more of them.
Social science has been trying to solve this problem of “deviancy training” for more than 20 years, says Graduate School of Social Work (GSSW) Assistant Professor Anamika Barman-Adhikari, PhD. She may have a solution.
Instead of randomly assigning youth to treatment groups — the typical practice — what if they were assigned in such a way that positive influences were maximized and negative influences were minimized? Using an artificial intelligence (AI) algorithm that considers factors such as how often individuals engage in substance use and other risky behaviors, Barman-Adhikari is partitioning groups to promote positive outcomes.
“We try to put a high-risk individual into a group that is less risk-taking and has more positive behaviors,” she explains. “They will be more likely to change their behaviors if they’re influenced by positive forces.”
Barman-Adhikari, GSSW doctoral student Daphne Brydon and colleagues at the University of Southern California — including Eric Rice, Milind Tambe and Phebe Vayanos at the Center for Artificial Intelligence in Society, and Viterbi School of Engineering doctoral student Aida Rahmatallabi — designed the algorithm using data collected in Los Angeles in 2013. The calculus is relatively simple, says Barman-Adhikari. “What are your social networks, and what risk behaviors do you engage in?”
When the researcher team tested the algorithm against the data, the simulation results were striking: Compared to randomly assigned groups, deviance training was reduced by almost 60 percent in AI-assigned groups.
“Regardless of the intervention,” Barman-Adhikari explains, “it is the composition of the group that changes the outcomes.”
The team is now embarking on a randomized controlled trial in collaboration with Urban Peak, a Denver-based nonprofit serving youth experiencing homelessness. Participants will complete an initial computer-based survey that asks them to identify their most consequential personal relationships over the last 30 days and then asks questions about each of those friends. Forty participants will be in the experimental, AI-composed treatment condition, and 40 will be in the control condition. Participants in both groups will receive the Project Towards No Drug Abuse intervention, which uses peer leaders to disseminate positive norms about substance abuse through role plays, group activities and weekly meetings.
“Urban Peak has seen this deviancy training process occurring in their own group-housing program, so they want to better configure youth into these groups so they can solve the problem,” Barman-Adhikari says.
Clinical testing will wrap up in November, and the research team is discussing how to take an AI-informed intervention to scale. “We want to make certain this is something we can scale up and that organizations can use it without additional burden,” says Barman-Adhikari, whose vision is to create an app-like program that organizations like Urban Peak can easily install and use.
AI holds broader promise, too. “We lack predictive models in social science,” says Barman-Adhikari, who Microsoft recently invited to India to discuss the role of AI in promoting social good. “We’re already using statistical and mathematical tools. AI learns and then automates the process so you don’t have to keep doing it over and over. AI will help social science intervention research by giving us very precise predictive tools so we can avoid mistakes we’ve made in the past.”
AI won’t be taking on the role of social workers, though. “Even when we get group configuration, we’ll take that back to Urban Peak social workers and validate those groups with human beings,” Barman-Adhikari says. “We are giving algorithm parameters to decide the most effective outcome given those parameters. Human beings still decide what’s important.”
In another study with DU colleagues, Barman-Adhikari studies the effects of technology and social media on homeless youth. Learn more.